ML Task Runner
The ML Task Runner is a specialized component that executes predefined machine learning tasks and workflows. It provides a structured way to run ML operations with configurable parameters and monitoring capabilities.
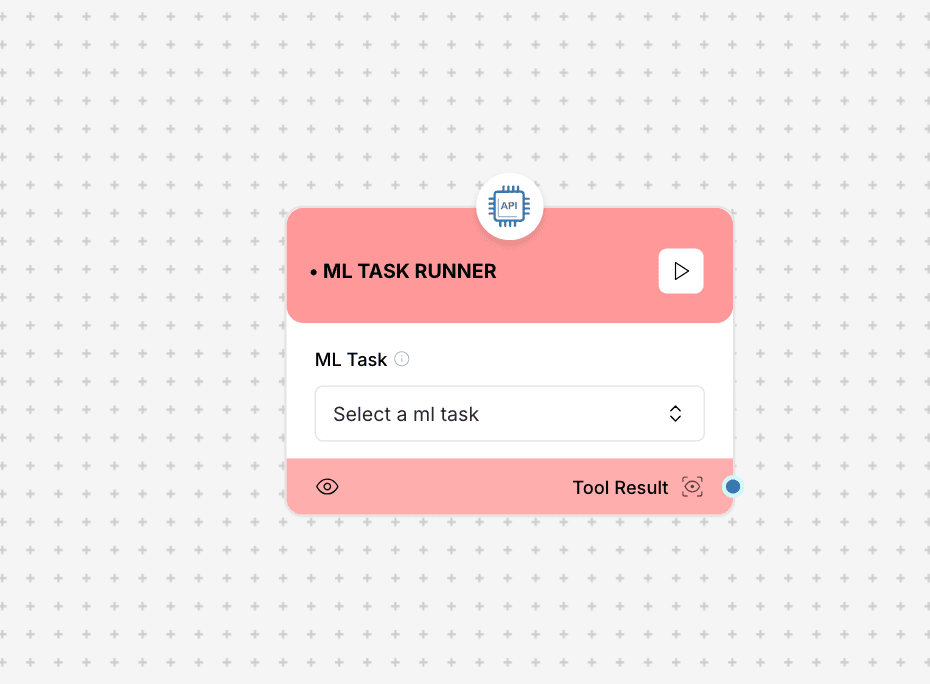
ML Task Runner interface and configuration
Usage Note: Select appropriate ML tasks based on your requirements. Ensure all necessary dependencies and resources are available before task execution.
Component Inputs
- ML Task: The machine learning task to execute
Example: Select from available ML tasks like "Training", "Inference", "Evaluation"
Component Outputs
- Tool Result: The outcome of the ML task execution
Example: Task completion status, metrics, or generated outputs
How It Works
The ML Task Runner orchestrates the execution of machine learning tasks through a systematic workflow. It handles task initialization, resource management, execution monitoring, and result collection.
Execution Process
- Task selection and validation
- Resource allocation
- Task initialization
- Execution monitoring
- Result collection
- Resource cleanup
Use Cases
- Model Training: Execute training workflows
- Inference Tasks: Run model predictions
- Evaluation Jobs: Perform model assessments
- Data Processing: Handle data preparation tasks
- Pipeline Execution: Run ML pipelines
Implementation Example
const mlTaskRunner = new MLTaskRunner({
mlTask: "ModelTraining",
parameters: {
modelType: "classification",
dataset: "training_data.csv",
epochs: 100
}
});
const result = await mlTaskRunner.execute();
// Output:
// {
// status: "completed",
// metrics: {
// accuracy: 0.95,
// loss: 0.05,
// trainingTime: "2h 30m"
// },
// artifacts: {
// modelPath: "/models/trained_model.h5",
// logsPath: "/logs/training.log"
// }
// }
Additional Resources
Best Practices
- Validate task requirements before execution
- Monitor resource utilization
- Implement proper error handling
- Keep task configurations versioned
- Regular task performance reviews