LLM Boolean Evaluator
The LLM Boolean Evaluator is a specialized component that evaluates text responses from language models and returns boolean (true/false) results based on specific criteria or conditions. It's particularly useful for validation checks and conditional logic in LLM workflows.
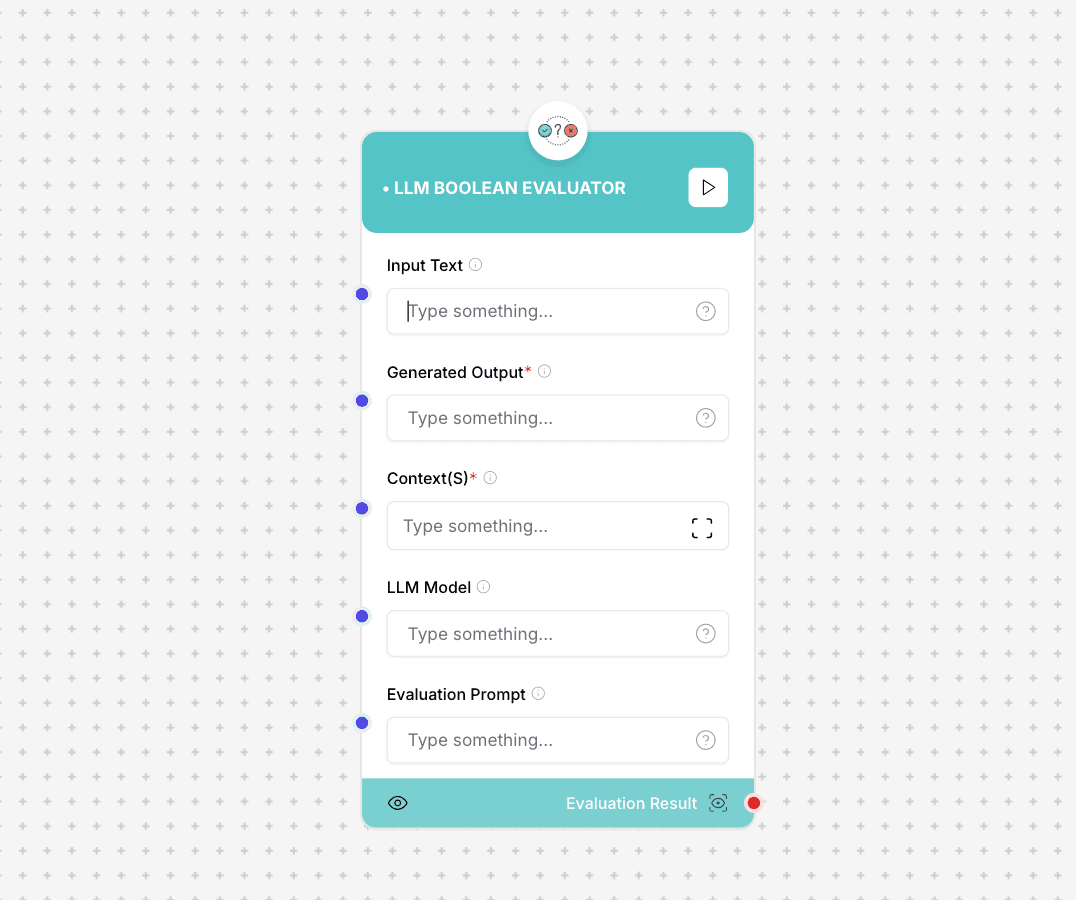
LLM Boolean Evaluator interface and configuration
Usage Note: Ensure that your evaluation criteria are clearly defined and unambiguous. The evaluator works best with well-defined conditions that can be definitively evaluated as true or false.
Component Inputs
- Input Text: The text to be evaluated
Example: "The statement to be verified"
- Generated Output: The model's response to evaluate
Example: "Response to check against criteria"
- Context(s): Additional context for evaluation
Example: "Relevant contextual information"
- LLM Model: The language model to use for evaluation
Example: "gpt-4", "claude-2"
- Evaluation Prompt: The specific condition to evaluate
Example: "Does this response contain a valid solution?"
Component Outputs
- Boolean Result: True/False evaluation result
Example: true or false
- Confidence Score: Confidence in the evaluation
Example: 0.95 (95% confidence)
- Explanation: Reasoning for the boolean result
Detailed explanation of the evaluation decision
How It Works
The LLM Boolean Evaluator processes inputs through a systematic evaluation pipeline to produce reliable true/false determinations. It uses context-aware analysis and specific evaluation criteria to make its decisions.
Evaluation Process
- Input and context analysis
- Criteria evaluation
- Boolean determination
- Confidence calculation
- Explanation generation
- Result validation
Use Cases
- Validation Checks: Verify if responses meet specific criteria
- Quality Control: Check if responses maintain quality standards
- Compliance Verification: Ensure responses follow guidelines
- Decision Making: Support automated decision processes
- Content Filtering: Determine if content meets specific conditions
Implementation Example
const booleanEvaluator = new LLMBooleanEvaluator({
inputText: "Is this solution secure?",
generatedOutput: "The solution implements encryption...",
context: "Security evaluation context",
llmModel: "gpt-4",
evaluationPrompt: "Does this response provide a secure solution?"
});
const result = await booleanEvaluator.evaluate();
// Output:
// {
// result: true,
// confidence: 0.92,
// explanation: "The solution implements proper security measures..."
// }
Best Practices
- Define clear evaluation criteria
- Provide comprehensive context
- Set appropriate confidence thresholds
- Validate results with test cases
- Monitor evaluation accuracy